Operation Point Selection
A Novel Learning-based Approach for Dual-Arm Robot Operation Point Selection
In the scenario of dual-arm robot collaborative manipulation, selecting the appropriate operation point can significantly influence the success rate and efficiency of the subsequent tasks. In our context, the so-called operation point, instead of manipulation points, refers to the three-dimensional positions in the workspace where the dual-arm robot performs its tasks. In this paper, we propose a novel learning-based approach for selecting the optimal operation point. Meanwhile, we design a specific measure to evaluate the selection of the dual-arm operation point. In the model training process, our approach enables the dual-arm robot to autonomously explore the workspace to try its best to find the most suitable operation point. To better validate our proposed approach, we designed a dual-arm robot platform that includes both the UR3 and AUBO i5 robotic arms, rather than two robotic arms with the same configuration. The experiment was conducted on the peg-in-hole assembly task. To efficiently train our model, we also established a corresponding simulation environment. The experimental results show that our approach has better performance in selecting operation points than traditional baseline approaches.
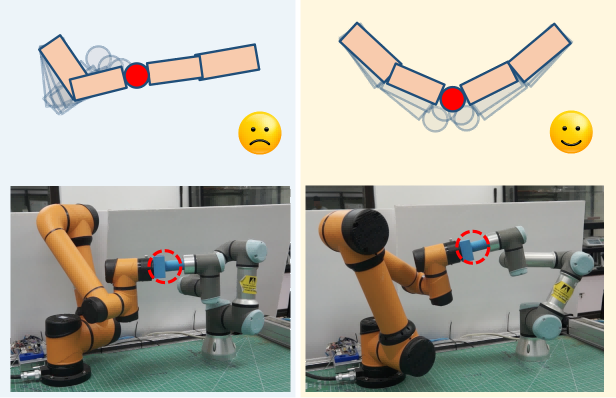
Video